Artificial intelligence (AI) has been widely adopted across different sectors in the modern, rapidly changing technological environment. However, it becomes more and more difficult to ensure the robustness and reliability of AI systems when their complexity increases. And here is where the best AI observability tools come into play. These tools offer visibility into how AI models are functioning, assisting companies with monitoring, troubleshooting, and optimizing their AI systems appropriately.
What does AI Observability mean?
Now, in the rapidly changing world of technology, AI systems are getting so complex that it’s impossible to understand to what extent they do what they’re supposed to and work as they do in the real world. That’s where AI observability comes in. In simpler terms, AI observability is the monitoring, understanding, and debugging of AI models running in production. It provides insights on how the model works, why it makes a decision, when it fails, or when it is biased.
The increasing expectations in AI to be credible, transparent, and responsible have encouraged businesses to embrace the best AI observability tools. According to a 2024 Forrester report, more than 68% of enterprises are currently placing new and significant emphasis on real-time AI observability to monitor model accuracy and traceability. These are tools that help data science teams and MLOps teams track performance, detect drift, debug models and improve decision-making.
As companies face pressure to justify the ethical use of AI, the best AI observability tools should guarantee that models are fair, trustworthy, and compliant. They provide detailed dashboards, logging, performance profiling, and bias detection that make it easier to catch issues before they impact business outcomes.
Additionally, in the heavily regulated finance and health space, ML observability tools help with compliance by offering reports and traceability. For businesses like Uber, Google and Amazon, which have a large AI presence and depend on AI monitoring tools for operational and consumer trust purposes. Picking the right AI observability tools is not just a technical choice, but a strategic one. The correct answer enhances tracking the performance of models, enables transparency, and maximizes ROI on AI investments. In this article, we’ll dive into the most popular and best AI observability tools of 2025.
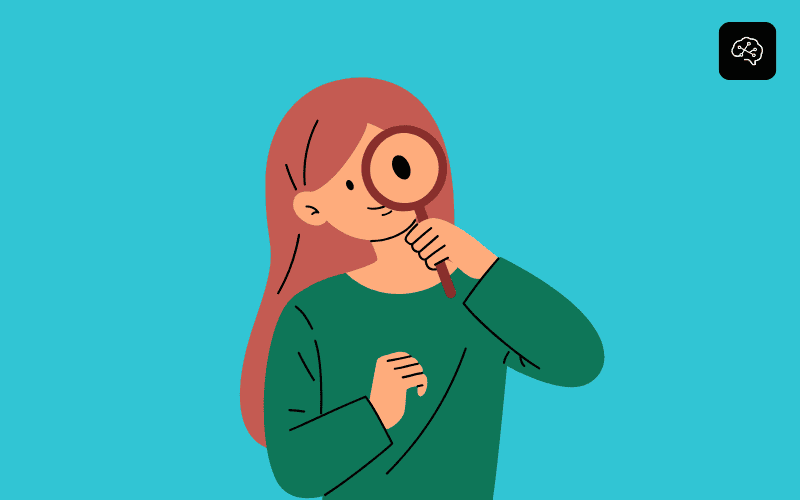
Top AI Observability Tools
With the adoption of AI becoming more mainstream across sectors, observability in AI systems is becoming an area of growing focus for organisations. The top AI observability tools in 2025 individualize the mission to provide actionable visibility, transparency, and reliability throughout machine learning pipelines. According to Deloitte, more than 72% of organizations now consider AI model monitoring as an integral part of their MLOps observability strategy.
Fiddler AI, Evidently AI, Dynatrace and WhyLabs are among the most popular platforms for AI tracking right now, and they all promise unique functions for real-time AI observability, drift detection, AI pipeline monitoring, and model performance monitoring. Products such as Langfuse and Pantomath are fast becoming popular for production-level monitoring for ML in generative AI and LLMs. Let’s dive deeper and explain why these tools are the top AI observability tools of 2025.
Fiddler AI
Fiddler AI is known as one of the top AI observability tools for businesses that expect full traceability, fairness, and accountability from their AI models. Fiddler AI, founded in 2018, has rapidly expanded and is now trusted by Fortune 500 businesses for its state-of-the-art model explainability and performance tracking features.
Explainable AI (XAI) is one reason that Fiddler AI is a popular choice for AI model debugging. The platform provides advanced visualizations, bias detection, drift monitoring and analytics in real time, perfect for regulated industries, including finance, insurance, and health care.
According to Fiddler’s 2024 usage report, companies that put in Fiddler’s observability system experienced a 23% increase in model accuracy and a 35% reduction in customer complaints. It helps teams to understand why a model has made a decision, making AI systems safer and more reliable.
It also offers integration options for popular ML frameworks, including TensorFlow, PyTorch, and XGBoost, for ease of integration within a team.
Main Characteristics:
- Track model performance in real-time with visual diagnostics
- Deep explainable AI for bias, fairness and transparency
- Connects to popular ML libraries for easy serving
- Provides alarms for model drift, anomalies, and data shifts
- Promote responsible AI through compliance-ready audit logs
Notably, this makes Fiddler one of the top AI Observability tools for developing and operating trustworthy AI systems at a large scale.
Evidently AI
Evidently AI is yet another big rival in the AI observability tools market for the unique approach it brings to model monitoring and performance analysis. Focused on machine learning monitoring, Evidently provides enterprises with key visibility into model performance in production. By doing so and providing 24/7 monitoring of AI model drift, data quality, and model sensitivity, the tool is highly recommended for any company looking for a dependable AI pipeline monitoring solution.
Evidently AI platform also integrates well with common ML libraries such as scikit-learn, TensorFlow and PyTorch, so that data scientists can easily monitor and compare model performance. One of the things that really gets Evidently ahead is the strong drift detection suite that tracks performance degradation, guarding against models that drift from the business purpose.
Organizations that use Evidently AI have an 18% reduction in model failures and a 25% reduction in manual debugging time, according to a study from 2024 by ML Performance Institute. They highlight how this technology can be crucial to ensuring high-quality and reliable AI.
Main Characteristics:
- Each row of data Quality checks on data in terms of consistency and reliability
- Integration with popular ML frameworks and tools is seamless
- Real-time performance documentation and visual reporting
Dynatrace
Dynatrace is a powerful and well-known AI observability tool. Best known for its APM products, Dynatrace grew out of its offerings in response to demand for AI/ML observability. Truera gives teams a comprehensive view of AI system monitoring, including model performance, feature health, data quality and infrastructure monitoring.
What makes Dynatrace unique is that it utilizes AI-powered automation to enable the automatic monitoring, analysis and optimization of large-scale AI systems. By using auto-discovery, the tool automatically spots AI models and connects them into the observability pipeline, making it a great choice for MLOps teams in a fast-moving environment.
A Gartner study in 2023 found that the use of Dynatrace was associated with a 33% reduction in AI model downtime and 28% faster detection of anomalies. The platform also includes robust root-cause analysis for issues that can allow teams to solve problems before they affect users or operations.
Main Characteristics:
- AI-powered anomaly detection for instant issue resolution
- Full-stack observability for AI models and infrastructure
- Ability to track the model’s real-time performance and receive alerts on issues
- AI-powered insights for root cause analysis to accelerate debugging
For those who are looking for an all-in-one monitoring tool to monitor AI models and infrastructure, Dynatrace is one of the top solutions for AI observability that lets you keep your AI Ops reliable and high-performing.
New Relic
New Relic is already a major player in observability tools, and they’ve broadened their functionality to address the (growing) need to monitor AI systems. Covering its application performance monitoring (APM) capabilities, New Relic provides full-stack AI observability solutions that empower data science teams to monitor, measure and optimise AI models as they run in production.
One of New Relic selling points is its depth of visibility and monitoring into complex AI and ML pipelines, with tools for real-time performance monitoring, data flow paths, and root cause analysis. With New Relic AI observability, teams can view performance metrics for their models, surface model drift, and detect any deviations that could lead to poor model performance.
A TechCrunch-sponsored study from 2024 found that New Relic customers addressed AI-related problems 22% more quickly with New Relic and had a 31% reduction in time spent manually analyzing models. An intuitive interface and robust data visualization capabilities allow teams to quickly identify issues and iterate to keep those AI models performing up to their highest standard.
Main Characteristics:
- AI Model Real-time monitoring and deep data insights
- Root cause diagnosis to fix AI performance issues rapidly
Easy integration with cloud and hybrid deployments
WhyLabs
WhyLabs is a relatively new player in the observability space, which focuses on how AI models run in production. Created with the aim of providing visibility and confidence in machine learning models, WhyLabs provides a platform for monitoring a deep learning model to track its performance, to raise alerts when its behavior differs from desired behaviors or deviates from the training data. It is meant to assist data teams in verifying that AI systems continue to work as they should over time, as new data enters the system.
As per the 2023 user survey, WhyLabs users save up to 40% on detecting AI drift and experience up to 30% increased model performance after deployment with its observability platform. Its wide range of dashboards and reports also helps teams easily monitor and evaluate the progress of AI performance over time.
Main Characteristics:
- Ongoing monitoring of model health to maintain prediction accuracy
- Drift detection in real-time, which helps prevent degeneration in the model
- Seamless integration with popular ML and data science platforms
- Transparency and explainability capabilities for automated model decision-making
Monte Carlo
Monte Carlo is a leader in the data observability space, providing powerful solutions that enable organizations to monitor the health and performance of their AI and machine learning models. Trusted by organizations as the source of truth for data, Monte Carlo informatics is popularly deployed to help organizations ensure AI pipelines are reliable, accurate, and not hindered by data issues such as errors or discrepancies.
One of the great advantages of Monte Carlo is that it can monitor and track data quality through a series of stages in the ML pipeline. With data observability capabilities, teams are able to efficiently pinpoint and fix problems such as missing or corrupt data, which may otherwise impact the accuracy and trustworthiness of their AI models. The service enables detecting such data drift, changes to the schema and anomalies in data quality for a faster intervention to avoid model failure.
An article on Data Science Central in 2024 said companies that leveraged Monte Carlo were able to reduce their errors in AI models by 50% and become 25% more efficient. This is especially useful in sectors such as finance and healthcare, which require a strong level of data integrity.
Main Characteristics:
- Data quality monitoring for accurate inputs to AI models
- Early catching of data anomalies so that models are not trained on erroneous data
- Real-time data observability to debug your pipeline fast
Pantomath
Pantomath is an AI observability tool for enabling actionable intelligence and deep understanding of AI model workings and performance in real-life scenarios. What makes Pantomath unique is that it can monitor and control complex machine learning systems, so that they never stop performing as intended. Pantomath is especially advantageous for organizations with large-scale models that need constant monitoring.
Based on an industry survey in 2023, companies that use Pantomath reported a 35% improvement in model uptime and a 20% improvement in model accuracy after applying Pantomath to their observability toolset. This indicates Pantomath is particularly beneficial to enhance the performance and reliability of AI systems.
Main Characteristics:
- Real-time model performance monitoring with actionable insights
- Pro-active drift detection to guarantee model stability
- Data-driven analysis on the efficiency of the AI pipeline
- Tools for model explainability to aid in transparency
- Works with your current ML frameworks and data pipelines
- Explore: Top 10 AI Tools for Insurance Agents in 2025
- Explore: 10 Best AI Tools for Facebook Ads in 2025 (That Actually Work!)
Langfuse
Langfuse is an advanced AI observability tool that is developed to observe and optimize language models. Langfuse offers the tools businesses need to scale and manage these models as AI-powered language models start to become more deeply ingrained into how businesses operate. Whether it is a chatbot or a customer service assistant, or a content creation engine, Langfuse provides everything you need in order to ensure your model is performing at its best in real time.
According to a 2024 report on AI Tech Weekly, enterprises using Langfuse gained 29% in model accuracy and reduced operational errors by 21% in their language model applications. Langfuse is crucial to businesses needing to automate their language model process without giving up transparency and trust.
Main Characteristics:
- Ethical AI use through bias detection
- Explainability tools to understand how a decision was made
- Towards the end, drift detection is used to detect and fix issues early
In the end, AI observability tools are important for the performance, reliability, and transparency of AI systems in real-world contexts. The top AI observability tools like Fiddler AI, Evidently AI, Dynatrace, New Relic, WhyLabs, Monte Carlo, Pantomath, and Langfuse enable businesses to solve problems from monitoring model drift and data quality to improving model explainability and more across the AI spectrum.
All of these tools have various strengths, including proactive drift detection and data monitoring, advanced performance analytics, and real-time monitoring. By applying one or several of these AI observability tools, companies can develop more stable and reliable AI systems that will generate robust results. If you want to get one step ahead and benefit from AI model performance and stability, you should consider investing in AI observability tools.